(ns foundations.computational.linguistics
(:require [reagent.core :as r]
[reagent.dom :as rd]
[clojure.zip :as z]
[clojure.pprint :refer [pprint]]
[clojure.string :refer [index-of]]
;[clojure.string :as str]
))
(enable-console-print!)
(defn log [a-thing]
(.log js/console a-thing))
(defn render-vega [spec elem]
(when spec
(let [spec (clj->js spec)
opts {:renderer "canvas"
:mode "vega"
:actions {
:export true,
:source true,
:compiled true,
:editor true}}]
(-> (js/vegaEmbed elem spec (clj->js opts))
(.then (fn [res]
(. js/vegaTooltip (vega (.-view res) spec))))
(.catch (fn [err]
(log err)))))))
(defn vega
"Reagent component that renders vega"
[spec]
(r/create-class
{:display-name "vega"
:component-did-mount (fn [this]
(render-vega spec (rd/dom-node this)))
:component-will-update (fn [this [_ new-spec]]
(render-vega new-spec (rd/dom-node this)))
:reagent-render (fn [spec]
[:div#vis])}))
;making a histogram from a list of observations
(defn list-to-hist-data-lite [l]
""" takes a list and returns a record
in the right format for vega data,
with each list element the label to a field named 'x'"""
(defrecord rec [category])
{:values (into [] (map ->rec l))})
(defn makehist-lite [data]
{
:$schema "https://vega.github.io/schema/vega-lite/v4.json",
:data data,
:mark "bar",
:encoding {
:x {:field "category",
:type "ordinal"},
:y {:aggregate "count",
:type "quantitative"}
}
})
(defn list-to-hist-data [l]
""" takes a list and returns a record
in the right format for vega data,
with each list element the label to a field named 'x'"""
(defrecord rec [category])
[{:name "raw",
:values (into [] (map ->rec l))}
{:name "aggregated"
:source "raw"
:transform
[{:as ["count"]
:type "aggregate"
:groupby ["category"]}]}
{:name "agg-sorted"
:source "aggregated"
:transform
[{:type "collect"
:sort {:field "category"}}]}
])
(defn makehist [data]
(let [n (count (distinct ((data 0) :values)))
h 200
pad 5
w (if (< n 20) (* n 35) (- 700 (* 2 pad)))]
{
:$schema "https://vega.github.io/schema/vega/v5.json",
:width w,
:height h,
:padding pad,
:data data,
:signals [
{:name "tooltip",
:value {},
:on [{:events "rect:mouseover", :update "datum"},
{:events "rect:mouseout", :update "{}"}]}
],
:scales [
{:name "xscale",
:type "band",
:domain {:data "agg-sorted", :field "category"},
:range "width",
:padding 0.05,
:round true},
{:name "yscale",
:domain {:data "agg-sorted", :field "count"},
:nice true,
:range "height"}
],
:axes [
{ :orient "bottom", :scale "xscale" },
{ :orient "left", :scale "yscale" }
],
:marks [
{:type "rect",
:from {:data "agg-sorted"},
:encode {
:enter {
:x {:scale "xscale", :field "category"},
:width {:scale "xscale", :band 1},
:y {:scale "yscale", :field "count"},
:y2 {:scale "yscale", :value 0}
},
:update {:fill {:value "steelblue"}},
:hover {:fill {:value "green"}}
}
},
{:type "text",
:encode {
:enter {
:align {:value "center"},
:baseline {:value "bottom"},
:fill {:value "#333"}
},
:update {
:x {:scale "xscale", :signal "tooltip.category", :band 0.5},
:y {:scale "yscale", :signal "tooltip.count", :offset -2},
:text {:signal "tooltip.count"},
:fillOpacity [
{:test "isNaN(tooltip.count)", :value 0},
{:value 1}
]
}
}
}
]
}))
(defn hist [l]
(-> l
list-to-hist-data
makehist
vega))
; for making bar plots
(defn list-to-barplot-data-lite [l m]
""" takes a list and returns a record
in the right format for vega data,
with each list element the label to a field named 'x'"""
(defrecord rec [category amount])
{:values (into [] (map ->rec l m))})
(defn makebarplot-lite [data]
{
:$schema "https://vega.github.io/schema/vega-lite/v4.json",
:data data,
:mark "bar",
:encoding {
:x {:field "element", :type "ordinal"},
:y {:field "value", :type "quantitative"}
}
})
(defn list-to-barplot-data [l m]
""" takes a list and returns a record
in the right format for vega data,
with each list element the label to a field named 'x'"""
(defrecord rec [category amount])
{:name "table",
:values (into [] (map ->rec l m))})
(defn makebarplot [data]
(let [n (count (data :values))
h 200
pad 5
w (if (< n 20) (* n 35) (- 700 (* 2 pad)))]
{
:$schema "https://vega.github.io/schema/vega/v5.json",
:width w,
:height h,
:padding pad,
:data data,
:signals [
{:name "tooltip",
:value {},
:on [{:events "rect:mouseover", :update "datum"},
{:events "rect:mouseout", :update "{}"}]}
],
:scales [
{:name "xscale",
:type "band",
:domain {:data "table", :field "category"},
:range "width",
:padding 0.05,
:round true},
{:name "yscale",
:domain {:data "table", :field "amount"},
:nice true,
:range "height"}
],
:axes [
{ :orient "bottom", :scale "xscale" },
{ :orient "left", :scale "yscale" }
],
:marks [
{:type "rect",
:from {:data "table"},
:encode {
:enter {
:x {:scale "xscale", :field "category"},
:width {:scale "xscale", :band 1},
:y {:scale "yscale", :field "amount"},
:y2 {:scale "yscale", :value 0}
},
:update {:fill {:value "steelblue"}},
:hover {:fill {:value "green"}}
}
},
{:type "text",
:encode {
:enter {
:align {:value "center"},
:baseline {:value "bottom"},
:fill {:value "#333"}
},
:update {
:x {:scale "xscale", :signal "tooltip.category", :band 0.5},
:y {:scale "yscale", :signal "tooltip.amount", :offset -2},
:text {:signal "tooltip.amount"},
:fillOpacity [
{:test "isNaN(tooltip.amount)", :value 0},
{:value 1}
]
}
}
}
]
}))
(defn barplot [l m]
(vega (makebarplot (list-to-barplot-data l m))))
; now, for tree making
;(thanks to Taylor Wood's answer in this thread on stackoverflow:
; https://stackoverflow.com/questions/57911965)
(defn count-up-to-right [loc]
(if (z/up loc)
(loop [x loc, pops 0]
(if (z/right x)
pops
(recur (z/up x) (inc pops))))
0))
(defn list-to-tree-spec [l]
""" takes a list and walks through it (with clojure.zip library)
and builds the record format for the spec needed to for vega"""
(loop [loc (z/seq-zip l), next-id 0, parent-ids [], acc []]
(cond
(z/end? loc) acc
(z/end? (z/next loc))
(conj acc
{:id (str next-id)
:name (str (z/node loc))
:parent (when (seq parent-ids)
(str (peek parent-ids)))})
(and (z/node loc) (not (z/branch? loc)))
(recur
(z/next loc)
(inc next-id)
(cond
(not (z/right loc))
(let [n (count-up-to-right loc)
popn (apply comp (repeat n pop))]
(some-> parent-ids not-empty popn))
(not (z/left loc))
(conj parent-ids next-id)
:else parent-ids)
(conj acc
{:id (str next-id)
:name (str (z/node loc))
:parent (when (seq parent-ids)
(str (peek parent-ids)))}))
:else
(recur (z/next loc) next-id parent-ids acc))))
(defn maketree [w h tree-spec]
""" makes vega spec for a tree given tree-spec in the right json-like format """
{:$schema "https://vega.github.io/schema/vega/v5.json"
:data [{:name "tree"
:transform [{:key "id" :parentKey "parent" :type "stratify"}
{:as ["x" "y" "depth" "children"]
:method {:signal "layout"}
:size [{:signal "width"} {:signal "height"}]
:type "tree"}]
:values tree-spec
}
{:name "links"
:source "tree"
:transform [{:type "treelinks"}
{:orient "horizontal"
:shape {:signal "links"}
:type "linkpath"}]}]
:height h
:marks [{:encode {:update {:path {:field "path"} :stroke {:value "#ccc"}}}
:from {:data "links"}
:type "path"}
{:encode {:enter {:size {:value 50} :stroke {:value "#fff"}}
:update {:fill {:field "depth" :scale "color"}
:x {:field "x"}
:y {:field "y"}}}
:from {:data "tree"}
:type "symbol"}
{:encode {:enter {:baseline {:value "bottom"}
:font {:value "Courier"}
:fontSize {:value 14}
:angle {:value 0}
:text {:field "name"}}
:update {:align {:signal "datum.children ? 'center' : 'center'"}
:dy {:signal "datum.children ? -6 : -6"}
:opacity {:signal "labels ? 1 : 0"}
:x {:field "x"}
:y {:field "y"}}}
:from {:data "tree"}
:type "text"}]
:padding 5
:scales [{:domain {:data "tree" :field "depth"}
:name "color"
:range {:scheme "magma"}
:type "linear"
:zero true}]
:signals [{:bind {:input "checkbox"} :name "labels" :value true}
{:bind {:input "radio" :options ["tidy" "cluster"]}
:name "layout"
:value "tidy"}
{:name "links"
:value "line"}]
:width w}
)
(defn tree-depth
"get the depth of a tree (list)"
[list]
(if (seq? list)
(inc (apply max 0 (map tree-depth list)))
0))
(defn tree
"plot tree using vega"
[list]
(let [spec (list-to-tree-spec list)
h (* 30 (tree-depth list))]
(vega (maketree 700 h spec))))
(def categories '(N V Adj Adv P stop))
(def vocabulary '(Call me Ishmael))
(defn logsumexp [& log-vals]
(let [mx (apply max log-vals)]
(+ mx
(Math/log2
(apply +
(map (fn [z] (Math/pow 2 z))
(map (fn [x] (- x mx))
log-vals)))))))
(defn flip [p]
(if (< (rand 1) p)
true
false))
(defn sample-categorical [outcomes params]
(if (flip (first params))
(first outcomes)
(sample-categorical (rest outcomes)
(normalize (rest params)))))
(defn score-categorical [outcome outcomes params]
(if (empty? params)
(throw "no matching outcome")
(if (= outcome (first outcomes))
(Math/log2 (first params))
(score-categorical outcome (rest outcomes) (rest params)))))
(defn normalize [params]
(let [sum (apply + params)]
(map (fn [x] (/ x sum)) params)))
(defn sample-gamma [shape scale]
(apply + (repeatedly
shape (fn []
(- (Math/log2 (rand))))
)))
(defn sample-dirichlet [pseudos]
(let [gammas (map (fn [sh]
(sample-gamma sh 1))
pseudos)]
(normalize gammas)))
(defn update-context [order old-context new-symbol]
(if (>= (count old-context) order)
(throw "Context too long!")
(if (= (count old-context) (- order 1))
(concat (rest old-context) (list new-symbol))
(concat old-context (list new-symbol)))))
(defn hmm-unfold [transition observation order context current stop?]
(if (stop? current)
(list current)
(let [new-context (update-context
order
context
current)
nxt (transition new-context)]
(cons [current (observation current)]
(hmm-unfold
transition
observation
n-gram-order
new-context
nxt
stop?)))))
(defn all-but-last [l]
(cond (empty? l) (throw "bad thing")
(empty? (rest l)) '()
:else (cons (first l) (all-but-last (rest l)))))
In this chapter we consider the maximum likelihood solution to the
parsing problem for HMMs/FSAs. Recall that the
Principle of Maximum Likelihood chooses
the single element of the hypothesis space—otherwise known as a
point estimate—which maximizes the probability of the data,
or the likelihood of the hypothesis. If our hypothesis space is given
by \(h \in H\), our data by \(d\) and our (log) likelihood function
is given by \(\mathcal{L}(h;d)\) then the principle of maximum
likelihood can be expressed as follows.
\[\DeclareMathOperator*{\argmax}{arg\,max}
\hat{h} = \underset {h \in H }\argmax \mathcal{L}(h;d)\]
The joint probability of a sequence of words and categories under a
Hidden Markov Model is given by
\[\Pr(w^{(1)},\dots,w^{(k)},c^{(1)},\cdots,c^{(k)})=\prod_{i=1}^k
\Pr(w^{(i)} \mid c^{(i)}) \Pr(c^{(i)} \mid
c^{(i-1)})\]
We seek to find a sequence of categories \(\hat{c}^{(1)},\cdots,\hat{c}^{(k)}\) which maximizes this expression.
\[\DeclareMathOperator*{\argmax}{arg\,max}
\hat{c}^{(1)},\cdots,\hat{c}^{(k)} = \underset {c^{(1)},\cdots,c^{(k)}
}\argmax \prod_{i=1}^k
\Pr(w^{(i)} \mid c^{(i)}) \Pr(c^{(i)} \mid c^{(i-1)}) \}\]
Note that each term in the expression above, depends on the preceding
category. Thus, in order to maximize the expression, we will need to
perform a search over all sequences of categories, of which there are
exponentially many. However, as was the case with computing the
marginal or forward probability of a string under an HMM, we can make
use of the conditional independence assumptions of the model to derive
a more efficient algorithm. The resulting algorithm is known as the
Viterbi algorithm.
Let’s define a function, \(\mathbf{vt}\) which returns the probability
of the sequence of categories that maximizes the likelihood of some
sequence of observed words and ends in state \(c^{(k)}\). This
probability is called the Viterbi score of the sequence. Note that
here we are using the \(\max\) function, rather than the \(\argmax\)
function.
\[\begin{align}\mathbf{vt}(w^{(1)},\cdots,w^{(k)}, c^{(k)}) &=& &
\underset {c^{(1)},\cdots,c^{(k-1)}}\max \Pr(w^{(1)},\dots, w^{(k)},
c^{(1)},\cdots, c^{(k-1)}, c^{(k)})\\
\end{align}\]
Importantly, in the expression above, the \(max\) ranges over all of
the states up to state \(c^{(k)}\) but not including it, since it is
fixed. We can use the conditional independence assumption of the HMM
to decompose the expression above into two parts. One which maximizes
the final transition to \(c^{(k)}\) and one which maximizes the
earlier state sequence.
\[\begin{align}
\mathbf{vt}(w^{(1)},\cdots,w^{(k)},c^{(k)})
&=&
\Pr(w^{(k)}|c^{(k)})
\underset {c^{(k-1)}}\max \{ \Pr(c^{(k)}|c^{(k-1)})
&
\underset {c^{(1)},\cdots,c^{(k-2)}}\max
\Pr(w^{(1)},\dots, w^{(k-1)},c^{(1)},\cdots,
c^{(k-2)},c^{(k-1)}) \}
\\
\mathbf{vt}(w^{(1)},\cdots,w^{(k)},c^{(k)})
&=&
\Pr(w^{(k)}|c^{(k)})
\underset {c^{(k-1)}}\max \{ \Pr(c^{(k)}|c^{(k-1)})
&
\mathbf{vt}(w^{(1)},\cdots,w^{(k-1)},c^{(k-1)}) \}
\end{align}\]
Thus, the Viterbi score can be computed efficiently in a way
analogous to the inside probability, just by replacing the sums in the
definition of the inside score with \(\max\).
From the perspective of the trellis, we simply store the score of the
best path into each node, rather than the sum probability of all
paths into the node.
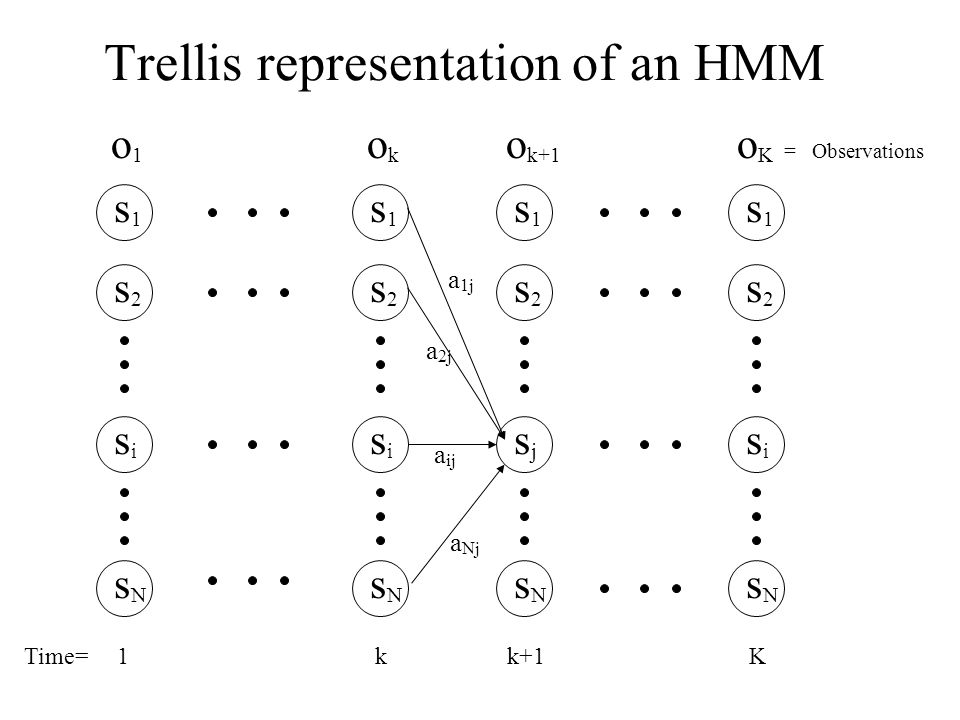
What does this look like
in code? First, let’s remind ourselves what the sampler for an HMM
looks like.
(def categories '(N V Adj Adv P stop))
(def vocabulary '(Call me Ishmael))
(def category->transition-probs
(memoize (fn [category]
(sample-dirichlet
(repeat (count categories) 1)))))
(defn sample-category [preceding-category]
(sample-categorical
categories
(category->transition-probs preceding-category)))
(def category->observation-probs
(memoize (fn [category]
(sample-dirichlet
(repeat (count vocabulary) 1)))))
(defn sample-observation [category]
(sample-categorical
vocabulary
(category->observation-probs category)))
(defn sample-categories-words [preceding-category]
(let [c (sample-category preceding-category)]
(if (= c 'stop)
'()
(cons
[c (sample-observation c)]
(sample-categories-words c)))))
(sample-categories-words 'start)
Given this sampler, we can now define a function which computes the
Viterbi score of a sequence and a state.
(defn viterbi-score [category sentence]
(if (empty? sentence)
0
(+
(apply
max
(map (fn [c]
(+ (viterbi-score c (all-but-last sentence))
(score-categorical
c categories
(category->transition-probs c))))
categories))
(if (= category 'stop)
0
(score-categorical
(last sentence) vocabulary
(category->observation-probs category))))))
(viterbi-score 'stop '(Call me Ishmael))
← 30 Parsing and Inference
32 Bayesian Parsing Methods →